Key Assumptions of the Dependent T-Test Explained
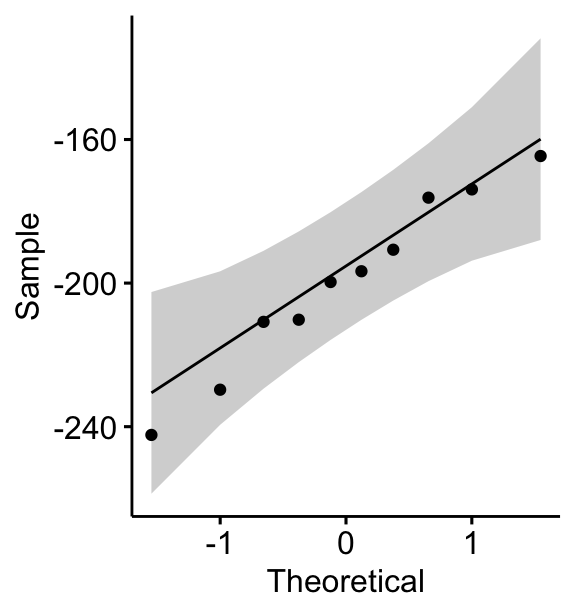
<!DOCTYPE html>
The dependent t-test is a powerful statistical tool used to compare the means of two related groups. It’s commonly applied in research scenarios where the same subjects are measured twice, such as before and after an intervention. Understanding its key assumptions is crucial for accurate results and valid conclusions. This post breaks down these assumptions, ensuring you can apply the test effectively in your analysis, whether for academic research or business decision-making. (dependent t-test assumptions, statistical analysis, paired t-test)
What is the Dependent T-Test?

The dependent t-test, also known as the paired t-test, is used to determine if there is a significant difference between the means of two related groups. Unlike the independent t-test, it focuses on paired observations, making it ideal for pre-post studies or matched-pairs designs. (paired t-test, pre-post studies, statistical significance)
Key Assumptions of the Dependent T-Test
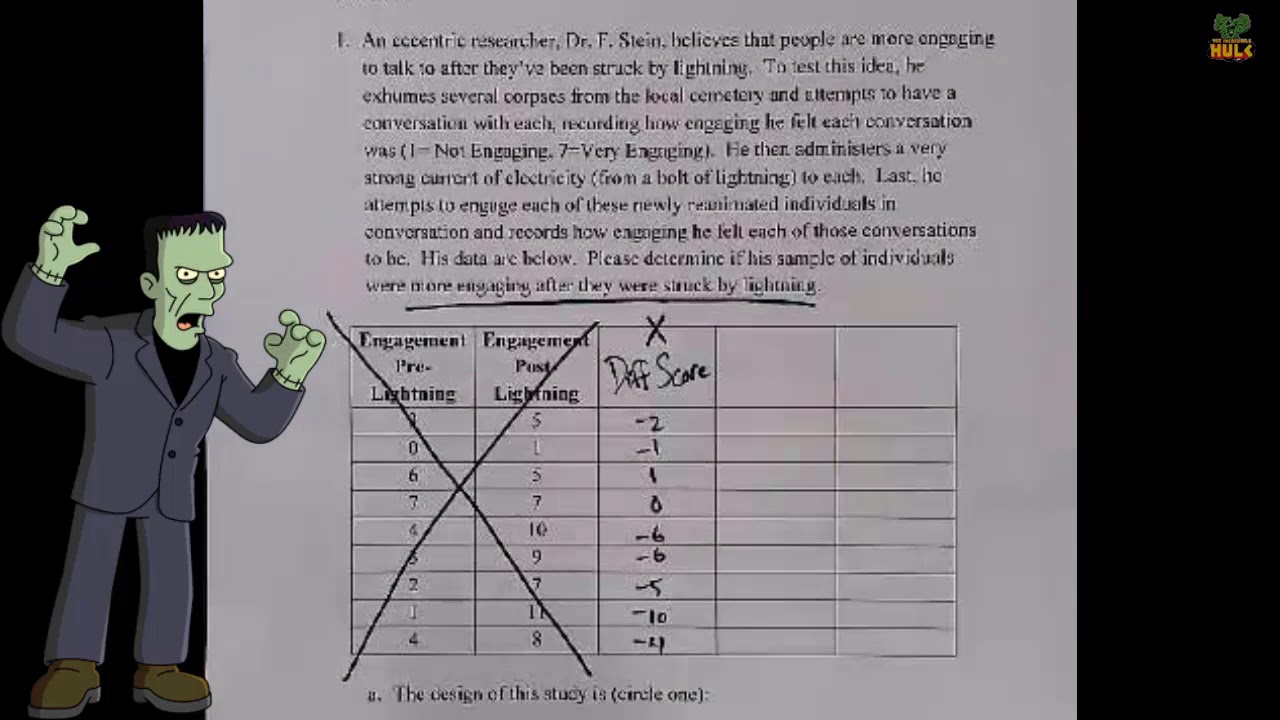
To ensure the validity of your results, the dependent t-test relies on several critical assumptions. Below, we explore each one in detail.
1. Paired Observations
The first assumption is that the data consists of paired observations. This means each observation in one group is directly related to an observation in the other group. For example, measuring the same individuals before and after a treatment. (paired observations, related groups)
2. Normality of Differences
The differences between the paired observations must follow a normal distribution. This assumption is crucial for the accuracy of the t-test. If the sample size is small, you can test for normality using methods like the Shapiro-Wilk test. (normal distribution, Shapiro-Wilk test)
3. Random Sampling
The data should be collected through random sampling to ensure that the sample is representative of the population. This reduces bias and increases the generalizability of your findings. (random sampling, representative sample)
📊 Note: Violating the random sampling assumption can lead to biased results and incorrect conclusions.
4. Independence of Observations
While the observations are paired, they must still be independent of each other. This means the difference between one pair should not influence the difference between another pair. (independence of observations)
How to Check These Assumptions
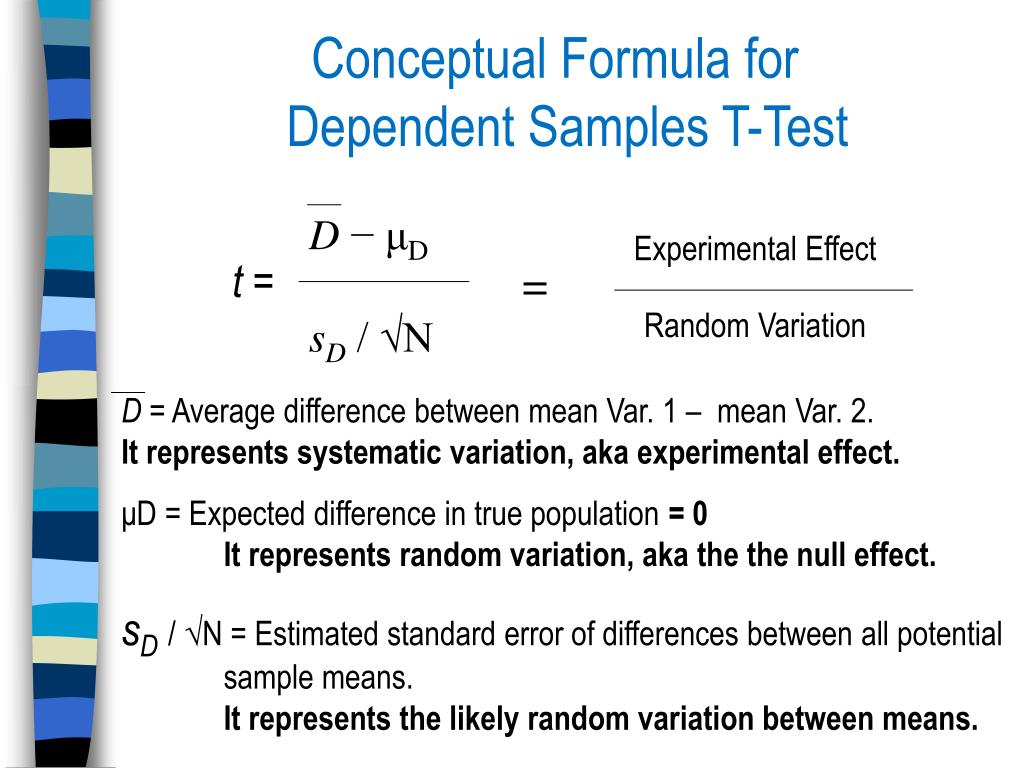
Before running a dependent t-test, it’s essential to verify these assumptions. Here’s a quick checklist:
- Confirm that your data consists of paired observations.
- Test for normality of differences using statistical tests or visual methods like Q-Q plots.
- Ensure your data is collected through random sampling.
- Verify the independence of observations.
When to Use the Dependent T-Test
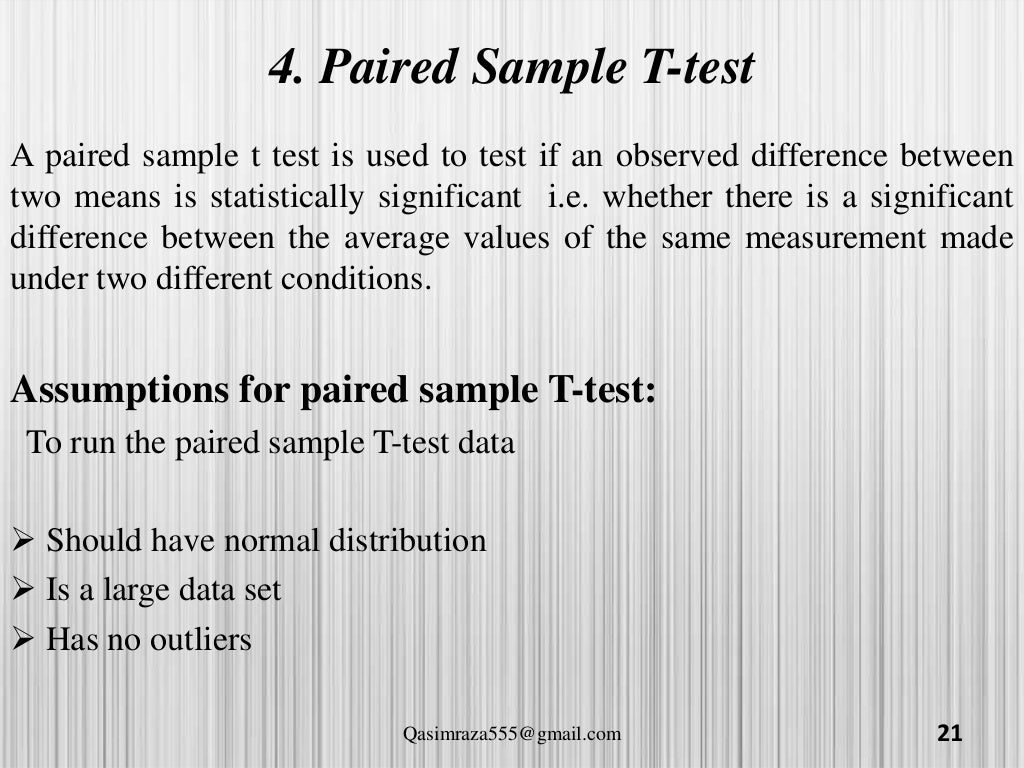
The dependent t-test is ideal for scenarios where you’re comparing related groups. Common applications include:
- Evaluating the effectiveness of a training program (pre-post measurements)
- Comparing test scores before and after an intervention
- Assessing changes in customer satisfaction over time
For businesses, this test can help measure the impact of marketing campaigns or product improvements. (marketing campaigns, product improvements)
Alternatives to the Dependent T-Test

If your data violates the assumptions of the dependent t-test, consider these alternatives:
- Wilcoxon Signed-Rank Test: A non-parametric alternative for non-normal data.
- Repeated Measures ANOVA: For more than two related groups.
💡 Note: Always choose the test that best fits your data and research question.
Understanding the key assumptions of the dependent t-test ensures your analysis is robust and reliable. By verifying these assumptions and selecting the appropriate test, you can draw accurate conclusions and make informed decisions. Whether you’re conducting academic research or analyzing business data, mastering these concepts will enhance your statistical toolkit. (dependent t-test assumptions, statistical analysis, paired t-test)
What is the main difference between dependent and independent t-tests?
+The dependent t-test compares paired observations, while the independent t-test compares two unrelated groups.
How do I test for normality in a dependent t-test?
+Use tests like the Shapiro-Wilk test or visualize data with Q-Q plots to check for normality of differences.
Can I use the dependent t-test if my data is not normally distributed?
+If your data violates normality, consider using the Wilcoxon Signed-Rank Test as a non-parametric alternative.