Unlocking Cancer Insights: Computational Biology Subfields Explained
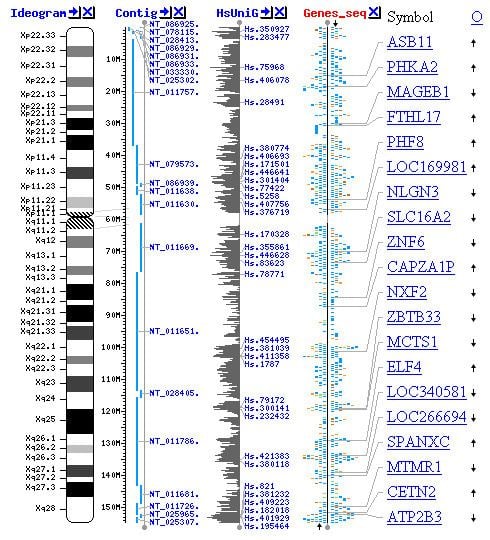
Cancer remains one of the most complex and challenging diseases to understand and treat. With advancements in technology, computational biology has emerged as a powerful tool in unlocking insights into cancer’s mechanisms, progression, and potential treatments. This blog explores the subfields of computational biology that are revolutionizing cancer research, offering both informative and commercial perspectives for readers. Whether you’re a researcher, healthcare professional, or simply curious about the intersection of biology and technology, this post is tailored to meet your needs.
Genomics and Bioinformatics: Decoding the Cancer Genome

Genomics and bioinformatics are at the forefront of computational biology’s contribution to cancer research. By analyzing vast datasets of genetic information, researchers can identify mutations, biomarkers, and pathways associated with cancer.
- Key Applications:
- Genome Sequencing: Identifying genetic mutations that drive cancer.
- Data Analysis: Using algorithms to interpret complex genomic data.
- Personalized Medicine: Tailoring treatments based on an individual’s genetic profile.
- Genome Sequencing: Identifying genetic mutations that drive cancer.
💡 Note: Advances in bioinformatics tools have significantly reduced the time and cost of genomic analysis, making it more accessible for cancer research.
Systems Biology: Understanding Cancer as a Complex System

Systems biology takes a holistic approach, modeling cancer as an interconnected network of genes, proteins, and cellular processes. This subfield helps researchers understand how disruptions in these networks lead to cancer.
- Key Techniques:
- Network Analysis: Mapping interactions between biological molecules.
- Mathematical Modeling: Simulating cancer progression and treatment responses.
- Integrative Omics: Combining genomics, proteomics, and metabolomics data.
- Network Analysis: Mapping interactions between biological molecules.
Subfield | Application in Cancer Research |
---|---|
Genomics | Identifying cancer-causing mutations |
Systems Biology | Modeling tumor microenvironments |
Machine Learning | Predicting treatment outcomes |

Machine Learning in Cancer Research: Predicting and Personalizing Treatments

Machine learning (ML) algorithms are transforming cancer research by analyzing large datasets to predict disease outcomes, identify potential drug targets, and personalize treatments.
- Applications:
- Predictive Modeling: Forecasting cancer recurrence or response to therapy.
- Drug Discovery: Accelerating the identification of effective cancer drugs.
- Imaging Analysis: Enhancing the accuracy of cancer diagnosis through AI-powered imaging.
- Predictive Modeling: Forecasting cancer recurrence or response to therapy.
🚀 Note: Machine learning is particularly valuable in handling the vast amounts of data generated in cancer research, enabling faster and more accurate insights.
Structural Biology and Molecular Modeling: Visualizing Cancer at the Molecular Level

Structural biology and molecular modeling allow researchers to visualize the 3D structures of proteins and molecules involved in cancer. This subfield is crucial for understanding how drugs interact with cancer cells.
- Key Contributions:
- Drug Design: Creating targeted therapies based on molecular structures.
- Protein Interactions: Studying how proteins contribute to cancer development.
- Virtual Screening: Identifying potential drug candidates computationally.
- Drug Design: Creating targeted therapies based on molecular structures.
Commercial Opportunities in Computational Biology

For commercial-intent visitors, computational biology offers numerous opportunities in the cancer research and healthcare industries. Companies specializing in bioinformatics software, AI-driven diagnostics, and personalized medicine are at the forefront of innovation.
- Market Trends:
- Rising demand for genomic sequencing services.
- Growth in AI-powered cancer diagnostics tools.
- Expansion of data analytics platforms for cancer research.
- Rising demand for genomic sequencing services.
Final Thoughts
Computational biology is reshaping our understanding of cancer, from decoding its genetic basis to predicting treatment outcomes. By leveraging subfields like genomics, systems biology, machine learning, and structural biology, researchers and companies are making strides in the fight against cancer. Whether you’re seeking informative insights or exploring commercial opportunities, computational biology offers a wealth of possibilities.
What is computational biology?
+Computational biology uses computational and statistical techniques to analyze biological data, particularly in understanding complex diseases like cancer.
How does machine learning help in cancer research?
+Machine learning analyzes large datasets to predict cancer outcomes, identify drug targets, and personalize treatments, accelerating research and improving patient care.
What are the commercial applications of computational biology in cancer research?
+Commercial applications include bioinformatics software, AI-driven diagnostics, personalized medicine, and data analytics platforms for cancer research.
cancer research,computational biology,genomics,machine learning,bioinformatics,personalized medicine,drug discovery,AI in healthcare.